The Psychometric Properties of the Montreal Cognitive Assessment (MoCA)
A Comprehensive Investigation
Abstract
Abstract. The Montreal Cognitive Assessment (MoCA) is a test assessing global cognition in older adults which is often used by researchers and clinicians worldwide, although some of its psychometric properties have yet to be established. We focus on three fundamental aspects: the factorial structure of the MoCA, its general factor saturation, and the measurement invariance of the test. We administered the MoCA to a large sample of Japanese older adults clustered in three cohorts (69–71-year-olds, 79–81-year-olds, and 89–91-year-olds; N = 2,408). Our results show that the test has an overall stable hierarchical factorial structure with a general factor at its apex and satisfactory general-factor saturation. We also found measurement invariance across participants of different ages, educational levels, economic status, and sex. This comprehensive investigation thus supports the idea that the MoCA is a valid tool to assess global cognition in older adults of different socioeconomic status and age ranges.
Introduction
The Montreal Cognitive Assessment (hereinafter MoCA; Nasreddine et al., 2005) is one of the most common tests for measuring global cognition and detecting potential global cognitive impairment in older adults. Administering the MoCA is quick (takes approximately 10 minutes), and the test can be used with both older adults (65- to 80-year-olds) and old-older adults (e.g., 80+-year-olds). The test is thus a useful and convenient instrument for studying cognitive function (and dysfunction). For these reasons, the MoCA is currently employed by researchers and clinicians worldwide, as testified to by the numerous citations of Nasreddine and colleagues’ article in the literature (more than 10,000 citations in Google Scholar, as of December 2019).
The MoCA was designed to measure a construct of interest, that is, the subject’s global cognition, making the MoCA total score a measure of this construct. Numerous validation studies showed that the MoCA possesses fair sensitivity and specificity (e.g., Fujiwara et al., 2010; Gil et al., 2015; Ziad Samir Nasreddine & Patel, 2016; Ozdilek & Kenangil, 2014; Yeung et al., 2014). Nonetheless, some fundamental psychometric properties of the items on the MoCA are still unclear. Specifically, their factorial structure, reliability, and measurement invariance have not been satisfactorily investigated so far. Without clear knowledge about these properties, any inferences based on the MoCA’s scores remain doubtful.
In the analysis of the factorial structure of test items, three cases are possible. First, only one latent general factor is present (i.e., unidimensionality); in this case, the test measures just one construct of interest with a certain degree of reliability. Second, more than one latent factor is estimated (i.e., multidimensionality), though no general factor is present; in this case, the total test score is not particularly meaningful because it does not refer to any general construct. Third, the factorial structure of the test is multidimensional, but at the same time all the test items correlate with each other, which suggests the presence of a general factor; in this case, the total test score is indeed a measure of the putative general factor. However, the reliability of the total score of the test cannot be estimated with an index such as Cronbach’s α. In fact, like any other total factor saturation index, α is not trustworthy when the assumption of unidimensionality is not met (Zinbarg et al., 2005). Rather, an index of general factor saturation – the proportion of the total score variance of a test accounted for by a single common factor (Reise et al., 2013) – is necessary to correctly evaluate the reliability of the test (e.g., McDonald’s ω and Revelle’s β; Zinbarg et al., 2006).
Whether the MoCA score reflects only one general factor (unidimensionality) or more factors (with or without a general factor) is still a matter of debate. Some studies simply postulated unidimensionality (e.g., Delgado et al., 2019; Nasreddine et al., 2005); other studies provided evidence that the items of the MoCA are substantially unidimensional (Freitas et al., 2015; Luo et al., 2019). However, these latter studies implemented generalized partial credit modeling (Thomas, 2011), which requires summing clusters of the items of the MoCA into seven subscores (i.e., the clusters indicated by Nasreddine et al., 2005). Although viable, this approach postulates, rather than tests, a specific structure in the data. Such a priori assumptions about the data of the MoCA structure were also employed with factor analysis (Coen et al., 2016; Duro et al., 2010). In these cases, the items of the MoCA were found to be multidimensional with no general factor, but the number of factors is unclear. Finally, other researchers adopted a more exploratory approach and highlighted the tendency of the items of the MoCA to converge toward a multidimensional structure with a general factor (Freitas et al., 2012). However, no index of general factor saturation is provided.
Finally, to date, there has been no thorough analysis of the measurement invariance of the MoCA. This gap in the literature is particularly concerning. Measurement invariance is a crucial property of any test because it concerns whether the total scores of the test have the same meaning under a set of different conditions (e.g., between the sexes and across participants of different ages). Without establishing measurement invariance, one cannot make any meaningful comparison across groups.
There are several hierarchically organized levels of measurement invariance (Millsap & Yun-Tein, 2004). The least restrictive one is configural invariance, which denotes whether the items of a test show the same factorial structure across conditions. Weak invariance (also referred to as metric invariance) assumes configural invariance and requires the same factor loadings across groups and thus establishes that the constructs measured by the total scores of the test are manifested in the same way across groups. Simply put, this condition tells us that responses on the items refer to the latent constructs with the same metric across groups. Strong invariance (also referred to as scalar invariance) assumes weak invariance and requires the same intercepts across groups. Finally, the most restrictive level is strict invariance, which requires both strong invariance and equal variances of items across groups. Strict invariance, therefore, provides information about how precisely the construct of interest is measured.
This study implements a systematic analytical strategy to address the above issues. First, we establish whether the items of the MoCA subtend either a unidimensional or multidimensional structure (with or without a general factor). Second, we use exploratory factor analysis (EFA) and confirmatory factor analysis (CFA) to determine the exact factorial structure of the MoCA. Third, we provide several reliability indexes for both general factor saturation and total factor saturation. Finally, we test for measurement invariance in four fundamental demographic variables – age, educational level, economic status, and sex – that may constitute confounding elements in the assessment of global cognition.
Methods
Participants
The study included a total of 2,408 Japanese older adults who were organized into three cohorts accordingly to age range (69–71, 79–81, and 89–91). The data were retrieved from the SONIC survey and referred to the baseline assessment. All details regarding the SONIC survey can be retrieved from Gondo et al. (2016).
Variables
MoCA
We used the data of the Japanese version of the MoCA (for more details, see Suzuki et al., 2015). As in Freitas et al.’s study, the 32 dichotomous (0/1) items of the test were used in the analyses.
Demographic Variables
We examined sex (male, female), age cohort (see above), education, and self-reported economic status of the participants. Education included three levels, indicating the highest educational degree achieved by the participant (primary/middle school, high school, and university/college education). The economic status of the participants included three levels as well (no financial leeway, some financial leeway, good financial leeway).
Analytical Approach
We employed a systematic strategy. First, we calculated the percentage of correct responses in each item of the MoCA. Second, we ran several tests of unidimensionality. Third, we ran an exploratory factor analysis (EFA) to investigate the factorial structure of the MoCA in a randomly selected subsample (Zinbarg et al., 2005). Fourth, the results of the EFA were tested with confirmatory factor analysis (CFA), which included all the observations not used in the EFA model. Finally, we tested measurement invariance of the MoCA score between the sexes, age cohorts, educational level, and self-reported economic status.
Results
Descriptive Statistics
The descriptive statistics are summarized in Table 1.
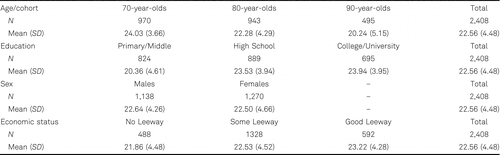
Data Preparation
We examined the mean correct response rate on each of the 32 items of the MoCA. To avoid estimation problems related to a ceiling effect (e.g., inflated item complexity in EFA), we excluded those items whose mean correct response was above 95% (n = 5). All subsequent analyses were thus performed with the remaining 27 dichotomous items of the MoCA. In addition, to control for any differences introduced by this technical choice, we replicated the analyses with all the 32 items. Only negligible differences were found (see the Supplemental Materials in https://osf.io/bcv4f/).
Dimensionality Tests
We first ran a unidimensionality check on a Rasch model (Drasgow & Lissak, 1983; Rasch, 1960). The analyses were performed with the ltm R package (R Core Team, 2017; Rizopoulos, 2006). This analysis showed evidence of multidimensionality (p < .010). We then ran a parallel analysis (Hayton et al., 2004) to establish the number of first-order factors with the psych R package (Revelle, 2017), using the tetrachoric correlation matrix and the weighted least squares (WLS) estimator. The parallel analysis estimated eight factors. The inspection of eigenvalues suggested the presence of a general factor (first eigenvalue = 6.40, second eigenvalue = 1.67).
Exploratory Factor Analysis
The previous analyses established that our data showed a multifactorial structure. First, following standard guidelines (Kyriazos, 2018), we randomly selected half of the participants (N = 1,204; calibration sample), while the other half of the sample was used in the CFA analysis (see below). We then ran a hierarchical EFA with seven first-order factors and assuming a general factor, as indicated by the parallel analysis. We performed the analyses with the psych R package. All items loaded onto the general factor (g). The seven subfactors corresponded approximatively to the seven subsets indicated by Nasreddine and colleagues (2005). Overall, the items thus exhibited a stable multifactorial structure. Table 2 summarizes the results of the EFA.
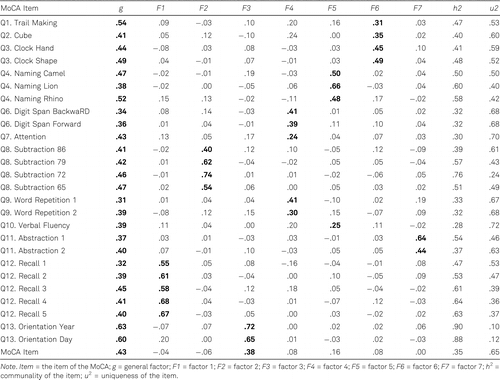
Confirmatory Factor Analysis
We built a hierarchical CFA model following the results of the EFA, using the other half of the sample. This model included seven first-order factors loading onto one second-order factor. Since the indicators were dichotomous, we used the WLSMV estimator. We performed these analyses with the lavaan and semTools R packages (Rosseel, 2015; Terrence et al., 2018).
We calculated the statistical power for not-close fit hypothesis testing (Kline, 2016). Assuming the null RMSEA = .050, alternative RMSEA = .010, the statistical power was more than adequate (> 99%) for global fit testing and rejection of false models (5% significance threshold). The CFA model exhibited good fit, χ2(317) = 453.776, p < .001, RMSEA = .019, and SRMR = .063, CFI = .970, and NCI = .972 (Hu & Bentler, 1999). The model estimated by the EFA analysis was thus confirmed by the CFA model in an independent sample.
Reliability Indexes
We calculated McDonald’s omega (ω) coefficients and Revelle’s beta (β) coefficient to measure the general factor saturation of the MoCA. The percentage of the reliable (i.e., not due to random error) variance – the ratio between omega hierarchical (ωh) and omega total (ωt) multiplied by 100 (Reise et al., 2013) – in the MoCA total scores accounted for by the general factor g was satisfactory (74%; ωh = .68 and ωt = .92). Revelle’s beta, which represents the minimum split-half reliability, was adequate too (β = .78). Total factor saturation indexes were similar to ωt (Cronbach’s α = .89 and Guttman’s λ6 = .91).
Measurement Invariance
Finally, we tested whether the items of the MoCA exhibited configural, weak, and strict invariance across the age groups, education level, sex, and economic status of the participants (strong invariance cannot be tested with dichotomous indicators). We performed these analyses on the whole sample (N = 2,408) with the semTools R package.
We ran the analyses according to the guidelines provided by Chen and colleagues (2005), Rudnev and colleagues (2018), and Wu and Estabrook (2016). First, we tested configural invariance by imposing the model’s threshold to be equal across groups. Second, we tested two types of weak invariance by imposing equality constraints to (1) the factor loading between first-order factors and the observed variables (i.e., the items of the test; Weak – 1st) and then (2) all the factor loadings (Weak – Full). Finally, we first tested strict invariance by applying equality constraints to the variances of the first-order factors (the variances of the indicators were fixed to 1 in the configural model; Wu & Estabrook, 2016).
We tested measurement invariance by inspecting the difference (Δ) in fit indexes in increasingly constrained models (e.g., configural vs. weak). The thresholds for model rejection were ΔRMSEA > .015, ΔSRMR > .030, ΔCFI < −.010, and ΔNCI < −.020 (Chen, 2007; Cheung & Rensvold, 2002). Measurement invariance was confirmed for all four demographic variables. None of the fit indexes reached its threshold for model rejection (maximum ΔRMSEA = +.001; ΔSRMR = +.005; ΔCFI = −.003; ΔNCI = −.006). Moreover, the chi-squared different test, whose Type I error rate is notoriously high, barely reached statistical significance only in one case (p = .044). Table 3 summarizes the results of this analysis.
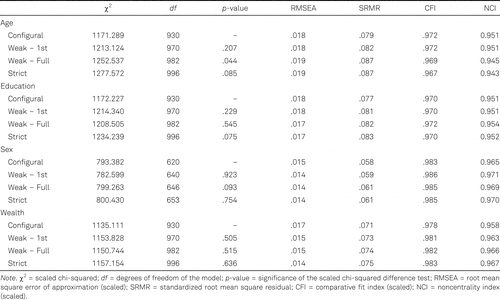
Discussion
This paper evaluates the psychometric properties of the items of the MoCA in a large sample of Japanese older adults. Specifically, we investigated (1) the dimensionality of the MoCA’s items, (2) their factorial structure, (3) their total factor saturation and general factor saturation, and (4) whether measurement invariance occurs across a set of fundamental demographic variables such as age, education, economic status, and sex. The analytical strategy implemented includes a set of exploratory methods (parallel analysis and EFA) and confirmatory methods (CFA and measurement invariance analysis). This choice allowed us to cross-validate the factorial structure of the items of the test in two independent samples without applying any a priori data structure (e.g., summing the items according to Nasreddine et al.’s categorization).
The results show that, overall, the MoCA is a valid tool for assessing global cognition in older adults. First, the presence of a general factor – along with 7 subfactors – indicates that the total score of the test is indeed a measure of global cognition. This factorial structure is verified in both the EFA and CFA models. Second, the reliability of the items of the MoCA is adequate in terms of both total factor saturation (α = .89 and λ6 = .91) and, most notably, general factor saturation (ωh/ωt = .74 and β = .78). Third, measurement invariance occurs is confirmed from the least restrictive model (configural invariance) to the most restrictive model (strict invariance) in all the demographic variables examined. This finding is of particular interest because no previous investigation has ever included a comprehensive measurement invariance analysis of the MoCA.
Furthermore, the present study may offer some insights regarding the inconsistent outcomes provided by the previous literature. As seen, measurement invariance analysis and general factor saturation have substantially remained untested. By contrast, the research on the MoCA’s dimensionality and factorial structure has been somewhat more abundant but has produced mixed results. Our investigation corroborates the assumption of a general factor made by several previous studies (Delgado et al., 2019; Freitas et al., 2015; Luo et al., 2019; Nasreddine et al., 2005). The only difference is that these studies report that the general factor is also the only latent dimension in the data (unidimensionality). The difference with our results, which suggest multidimensionality in the MoCA’s items, simply stems from a methodological choice. In fact, we did not impose any a priori constraint to the data (e.g., summing sets of items) that would conceal the hierarchical factorial structure of the items of the test.
Conclusions
The present study reports a comprehensive psychometric analysis of the MoCA. The test shows a stable hierarchical factorial structure, satisfactory general factor saturation, and measurement invariance in key demographic variables. The MoCA thus proves to be a reliable tool for assessing global cognition in older adults.
We gratefully thank Fred Oswald and Yves Rosseel for their assistance with the analyses. We also thank Fernand Gobet for providing comments on an earlier draft.
References
2007). Sensitivity of goodness of fit indexes to lack of measurement invariance. Structural Equation Modeling, 13, 464–504. https://doi.org/10.1080/10705510701301834
(2005). Testing measurement invariance of second-order factor models. Structural Equation Modeling, 12, 471–492. https://doi.org/10.1207/s15328007sem1203_7
(2002). Evaluating goodness-of-fit indexes for testing measurement invariance. Structural Equation Modeling, 9, 233–255. https://doi.org/10.1207/S15328007SEM0902_5
(2016). Strengths and limitations of the MoCA for assessing cognitive functioning: Findings from a large representative sample of Irish older adults. Journal of Geriatric Psychiatry and Neurology, 29, 18–24. https://doi.org/10.1177/0891988715598236
(2019). Validation of the Spanish-language version of the Montreal Cognitive Assessment test in adults older than 60 years. Neurología (English Edition), 34, 376–385. https://doi.org/10.1016/J.NRLENG.2018.12.008
(1983). Modified parallel analysis: A procedure for examining the latent dimensionality of dichotomously scored item responses. Journal of Applied Psychology, 68, 363–373. https://doi.org/10.1037/0021-9010.68.3.363
(2010). Validation studies of the Portuguese experimental version of the Montreal Cognitive Assessment (MoCA): Confirmatory factor analysis. Journal of Neurology, 257(5), 728–734. https://doi.org/10.1007/s00415-009-5399-5
(2015). Scaling cognitive domains of the Montreal Cognitive Assessment: An analysis using the partial credit model. Archives of Clinical Neuropsychology, 30, 435–447. https://doi.org/10.1093/arclin/acv027
(2012). Construct validity of the Montreal Cognitive Assessment (MoCA). Journal of the International Neuropsychological Society, 18, 242–250. https://doi.org/10.1017/S1355617711001573
(2010). Brief screening tool for mild cognitive impairment in older Japanese: Validation of the Japanese version of the Montreal Cognitive Assessment. Geriatrics and Gerontology International, 10, 225–232. https://doi.org/10.1111/j.1447-0594.2010.00585.x
(2015). Validation of the Montreal Cognitive Assessment (MoCA) in Spanish as a screening tool for mild cognitive impairment and mild dementia in patients over 65 years old in Bogotá, Colombia. International Journal of Geriatric Psychiatry, 30, 655–662. https://doi.org/10.1002/gps.4199
(2016).
(SONIC Study: A longitudinal cohort study of the older people as part of a centenarian study . In P. NancyEd., Encyclopedia of geropsychology (pp. 1–10). Springer Science. https://doi.org/10.1007/978-981-287-080-32004). Factor retention decisions in exploratory factor analysis: A tutorial on parallel analysis. Organizational Research Methods, 7, 191–205. https://doi.org/10.1177/1094428104263675
(1999). Cutoff criteria for fit indexes in covariance structure analysis: Conventional criteria versus new alternatives. Structural Equation Modeling, 6, 1–55. https://doi.org/10.1080/10705519909540118
(2016). Principles and practice of structural equation modeling, The Guilford Press.
(2018). Applied psychometrics: The 3-faced construct validation method, a routine for evaluating a factor structure. Psychology, 9, 2044–2072. https://doi.org/10.4236/psych.2018.98117
(2020). Applying item response theory analysis to the Montreal Cognitive Assessment in a low-education older population. Assessment, 27, 1416–1428. https://doi.org/10.1177/1073191118821733
(2004). Assessing factorial invariance in ordered-categorical measures. Multivariate Behavioral Research, 39, 479–515. https://doi.org/10.1207/S15327906MBR3903_4
(2005). The Montreal Cognitive Assessment, MoCA: A brief screening tool for mild cognitive impairment. Journal of the American Geriatrics Society, 53, 695–699. https://doi.org/10.1111/j.1532-5415.2005.53221.x
(2016). Validation of Montreal Cognitive Assessment, MoCA, alternate French versions. Canadian Journal of Neurological Sciences, 43, 665–671. https://doi.org/10.1017/cjn.2016.273
(2014). Validation of the Turkish version of the Montreal Cognitive Assessment Scale (MoCA-TR) in patients with Parkinson’s disease. Clinical Neuropsychologist, 28, 333–343. https://doi.org/10.1080/13854046.2014.881554
(2017). R: A language and environment for statistical computing, Foundation for Statistical Computing, Vienna. http://www.R-project.org/
. (1960). Probabilistic models for some intelligence and achievement tests, Danish Institute for Educational Research. https://doi.org/10.1016/S0019-9958(61)80061-2
(2013). Scoring and modeling psychological measures in the presence of multidimensionality. Journal of Personality Assessment, 95, 129–140. https://doi.org/10.1080/00223891.2012.725437
(2017). psych: Procedures for personality and psychological research (R package), Northwestern University Press.
(2006). ltm: An R package for latent variable modeling. Journal of Statistical Software, 17, 1–25.
(2015). lavaan: An R package for structural equation modeling. Journal of Statistical Software, 48, 1–36. https://doi.org/10.18637/jss.v048.i02
(2018). Testing measurement invariance for a second-order factor: A cross-national test of the alienation scale. Methods, Data, Analyses, 12, 47–76. https://doi.org/10.12758/mda.2017.11
(2015). One-year change in the Japanese version of the Montreal Cognitive Assessment performance and related predictors in community-dwelling older adults. Journal of the American Geriatrics Society, 63, 1874–1879. https://doi.org/10.1111/jgs.13595
(2018). semTools: Useful tools for structural equation modeling. R package version 0.5–1. https://cran.r-project.org/package=semTools
(2011). The value of item response theory in clinical assessment: A review. Assessment, 18, 291–307. https://doi.org/10.1177/1073191110374797
(2016). Identification of confirmatory factor analysis models of different levels of invariance for ordered categorical outcomes. Psychometrika, 81, 1014–1045. https://doi.org/10.1007/s11336-016-9506-0
(2014). A validation study of the Hong Kong version of Montreal Cognitive Assessment (HK-MoCA) in Chinese older adults in Hong Kong. Hong Kong Medical Journal, 20, 504–510. https://doi.org/10.12809/hkmj144219
(2005). Cronbach’s α, Revelle’s β and McDonald’s ωh: Their relations with each other and two alternative conceptualizations of reliability. Psychometrika, 70, 123–133. https://doi.org/10.1007/s11336-003-0974-7
(2006). Estimating generalizability to a latent variable common to all of a scale’s indicators: A comparison of estimators for ωh. Applied Psychological Measurement, 30, 121–144. https://doi.org/10.1177/0146621605278814
(