Identifying Determinants of Neuro-Enchancement Substance Use in Students
Application of an Integrated Theoretical Model
Abstract
Abstract.Background: Use of Neuro-Enhancement Substances (NES) such as prescription drugs, illicit drugs, or alcohol to improve cognition, prosocial behavior, and performance is increasing among students. Aims: The study applied a multi-theory, integrated theoretical model to identify motivational and social cognition determinants of NES use among students. Methods: A prospective longitudinal design was adopted with 306 high school (66.8% female; Mage = 17.31 years, SD = 0.93) and 692 university (70.5% female; Mage = 24.97 years, SD = 6.64) students. They completed measures of motivation, social cognition constructs, and planning with respect to studying behavior and NES use. Results: Well-fitting structural equation models indicated the pervasive influence of autonomous motivation, attitudes, subjective norms, and perceived behavioral control for studying, as well as of attitudes, subjective norms, and perceived behavioral control for NES use. Inclusion of past NES use increased explained variance in NES use and attenuated model effects, but the pattern of effects remained. Multi-group analyses indicated consistency in the pattern model effects across high school and university students. Limitations: The study findings might not be generalizable, as student samples were not randomly recruited. Furthermore, NES use only relied on self-report, and its assessment did not consider different NES substances. Finally, there was no assessment of implicit attitudes and habits toward studying and NES use. Conclusions: Findings extend prior literature by demonstrating the integrated guiding view that students’ motivation and beliefs about studying influence their beliefs about and use of NES. Furthermore, the findings provide starting points for interventions targeting the reduction in NES use.
The consumption of neuro-enhancement substances (NES) refers to the use of prescription drugs, illicit drugs, or alcohol to improve cognition, mood, or prosocial behavior (Maier & Schaub, 2015). The “United Nations Office on Drugs and Crime” guidelines (UNODC, 2011) recognize the rise in the use of these substances by school, college, and university students to improve their academic performance. Research suggests that the students’ use of NES has become increasingly common in many countries, with widespread use in the United States (e.g., Dussault & Weyandt, 2013), Canada (e.g. Kudlow et al., 2013), Australia (e.g., Lucke et al., 2018), Brazil (De Oliveira Cata Preta et al., 2020), and Europe (e.g., Majori et al., 2017).1 For instance, NES use among German students increased from 4% to 7% (Maier et al., 2013). Students report using NES primarily to increase motivation, vigilance, concentration, productivity, learning time, and mood (e.g., Maier et al., 2015). However, NES research findings are not equivocal and often suggest that NES effects on cognitive functioning can be attributed to placebo effects (Franke et al., 2014; Repantis et al., 2010). NES use might lead to several side effects. For example, the use of prescribed methylphenidate is linked to headache, tachycardia, and nervousness (Caplan et al., 2007; Repantis et al., 2010), and the deleterious consequences of misuse of drugs like alcohol or cannabis are well documented (Maier & Schaub, 2015). Even excessive use of “soft enhancers” can have detrimental side effects, such as short-term tachycardia, hypertonia, and bronchial dilatation (Franke et al., 2014). Given these potential health risks, authorities have become increasingly concerned with NES use by students and have issued guidelines on their control and regulation (Greely, 2008).
Efforts to control NES use require a comprehensive understanding of the determinants of the motives for NES use. Previous research has suggested that the decision to use NES in academic contexts can be considered a goal-directed and intentional behavior (Zelli et al., 2015). Furthermore, research focusing on belief systems has shown that students using prescription drugs without a diagnosis hold stronger views about the approval of significant others (subjective norms; SN) and demonstrate weaker perceived behavioral control (PBC) than do students with a specific diagnosis (Judson & Langdon, 2009). Finally, other studies have focused on the relation between academic self-efficacy and NES consumption (Verdi et al., 2016); however, such research is relatively sparse and highly descriptive.
An Integrated Theoretical Approach to Identify Behavioral Determinants
The examination of the determinants of NES use is highly valuable as it may assist in identifying potentially modifiable targets for interventions and in minimizing health risks and adverse consequences of NES use. One potential research avenue is the application of theories of motivation and social cognition, which not only enable the identification of determinants but also that of processes by which those determinants may relate to behavior (Hagger et al., 2017).
The present study adopted a multi-theory, integrated approach to identify the determinants and processes of NES use. The promise of the integrated approach is that it encompasses multiple constructs representing key determinants and processes. Provided there is a clear conceptual basis and empirical precedent, such integration maximizes the comprehensiveness of explanation of outcomes, it assists in addressing shortcomings of single theories, and it provides a means to represent different processes determining behavior (Hagger, 2009; Hagger & Hamilton, 2018; McMillan & Conner, 2007; Montaño & Kasprzyk, 2015).
Our integrated model draws its hypotheses from two leading theories of motivated action, the self-determination theory (SDT; Ryan & Deci, 2000) and the theory of planned behavior (TPB; Ajzen, 1991). SDT makes the distinction between two qualities of motivation: autonomous motivation presumes that one engages in behavior for personally endorsed reasons and out of a sense of choice and volition, and controlled motivation which reflects acting for externally-references reasons such as to gain a reward or external approval or to avoid punishment, guilt, or shame. Research has consistently shown that autonomous motivation is more likely to lead to behavioral persistence as well as to adaptive outcomes, whereas controlled motivation leads to persistence only as long as the reinforcing contingencies are present and tends to lead to non-adaptive outcomes (Chan & Hagger, 2012; Hagger et al., 2014; Ng et al., 2012). According to this type of integrated model (Chan et al., 2020; Hagger & Chatzisarantis, 2009), autonomous motives promote behavioral persistence, as individuals strategically align their beliefs about future behavioral performance with their motives, consistent with the original premise of the theory (Deci & Ryan, 1985, 2000).
Social cognition theories can model this process. Sets of beliefs from the TPB have been employed as mediators of the effects of autonomous motives on behaviors. Individuals citing autonomous reasons for adopting behavioral actions tend to hold positive attitudes about the behavior, expect that significant others would support their actions, and feel in control of the behavior. Such beliefs are proposed by scholars as the immediate antecedents of intention to perform the behavior in the future, and, therefore, forming such beliefs is strategically important to execute the behavior. Beliefs have utility in enabling individuals to act on their autonomous motives.
The integrated model in general, and the mediation of autonomous motives on behavior through beliefs and intentions, more specifically, have been supported in numerous studies (e.g., Chan et al., 2020; Hagger & Chatzisarantis, 2009; Jacobs et al., 2011). The model has also demonstrated efficacy as a means to guide interventions (e.g., Hagger & Luszczynska, 2014).
Models integrating hypotheses from SDT and the TPB also have recognized the consistently imperfect relation linking intention and behavior, often referred to as the “intention-behavior gap” (Hagger & Chatzisarantis, 2014; Orbell & Sheeran, 1998). This means that individuals do not always “follow through” on their intentions, as evidenced by studies in which behavioral variance tends to range between 20% and 30%. Existing dual-phase models of behavior propose that motivation or intention are necessary but not sufficient conditions for behavioral enactment and propose that intention needs to be augmented with plans for intention to be enacted (Gollwitzer & Brandstätter, 1997; Keller et al., 2020). For example, Gollwitzer (1999) suggested that specific types of plans, known as if-then plans or “implementation intentions,” assist individuals in linking appropriate environmental contingencies (e.g., cues) with the intended behavior, assisting in the recall and efficient enactment of the behavior. Such plans assist individuals in intention enactment and serve to moderate the intention-behavior relation. This moderation hypothesis has been added to existing integrated models to form a more comprehensive description of the determinants and processes that lead to behavior (Hagger & Chatzisarantis, 2014).
The Present Study
Our hypothesized integrated model might assist research focusing on the motivational determinants of NES use by students. The guiding model is diagrammed in Figure 1 and the online supplemental materials (https://osf.io/ngcas/, Table A: Summary of Hypothesized Direct and Indirect Effects). Concerning academic performance, indicated by success on assignments and in exams, students who are autonomously motivated to study are unlikely to view NES as necessary and may actively avoid taking such substances. Moreover, students who are autonomously motivated might personally value studying, as it serves the internalized goal of learning and advancing knowledge, with resultant good grades as indicators of their progress. Such students are likely to form beliefs and intentions to study in the future. They may hold attitudes, SN, and PBC beliefs that do not endorse NES use. In contrast, students with controlled motivation may view studying only to set good grades. In the absence of personal goals to learn and progress their knowledge, these students may seek any means to enhance their performance, as the outcome is viewed as the sole goal, and in the end, might view NES use as a viable proposition.
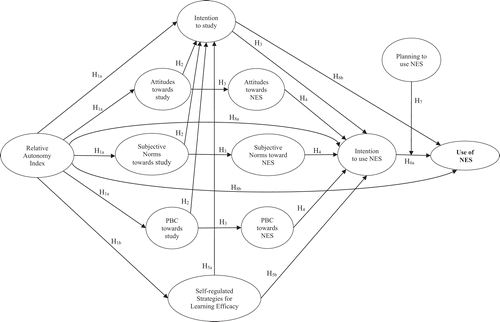
Autonomous motivation toward studying can thus be highly functional and adaptive in that it likely catalyzes alignment of beliefs toward studying and minimizes the chances of endorsing beliefs and intentions about NES use. Although autonomous motives to study might be inversely related to intention to use NES, planning is likely to be important to students enacting their NES use intentions. Implementation intentions are proposed to assist in the conversion of intention into behavior.
Based on the above considerations, the present study focused on the general mediation hypothesis that motivational states toward studying influence students’ possible use of NES by influencing students’ beliefs and intentions about studying, which in turn shape students’ beliefs, intentions, and planning about NES use. The current study thus proposes a sort of “nested” set of relations by which students’ specific beliefs, intentions, and possible use of NES at least partly depend upon (i.e., are nested within) students’ motivational states and beliefs about the broader behavioral domain of studying.
The study relied on data collected from both high school and university students, with behavioral data collected on two separate occasions over the course of several months. This longitudinal assessment permitted the examination of the strength of effects after controlling for the stability in students’ NES use which, being a proxy of students’ habits and previous decision making, may empirically attenuate the hypothesized effects (Hagger et al., 2016, 2017, 2018; Hagger et al., 2018). The present study also evaluated the “invariance” of the guiding model, that is, the general hypothesis that the relations would empirically hold in both high school and university students. Should invariance be found, it would provide strong evidence for the generalizability of the motivational determinants and cognitive processes guiding NES use among students.
Method
Procedure and Sampling
The study adopted a prospective design with two data collection occasions separated by four months, and it relied on data collected on two convenience samples of high school and university students. This choice was made to maximize the generalizability of the hypotheses tested and diagrammed in the guiding model of Figure 1. High school students were enrolled in a large urban district high school, and we recruited only those students who were enrolled in the last three school years. University students were from a large state university, and we included all students who regularly attended the university teaching courses. For the high school sample, data were collected from January (first wave) to April 2017 (second wave). For the university sample, data were collected from December 2016 (first wave) to March 2017 (second wave). These periods correspond to an intense study time, given high school or university exams. Overall, participants were predominantly females, and in the university student sample, there was a relatively large age range due primarily to nearly 20 students who were older than 45 years.
In particular, 306 high school students (66.8% female; Mage = 17.31 years, SD = 0.93, range = 16–19) and 692 university students (70.5% female; Mage = 24.97 years, SD = 6.64, range = 18–58) provided data on both occasions. The drop-out rate across the two data collection occasions was 11% (N = 34) in the high school sample and 15% (N = 105) in the university sample. Further sample characteristics (e.g., academic years, type of faculty) are available in the online supplemental materials (https://osf.io/ngcas/, Appendix A). The first assessment provided self-report data on the model’s psychological constructs and past NES use over the previous four months, and the second assessment provided self-report data on NES use since the first assessment. High school students were recruited during school days and provided written consent for participation. If minors, parents provided the consent. While high school students provided their data via paper-and-pencil questionnaires, university students gave their consent at the outset of a web-based questionnaire survey. The study was approved by the Ethics Review Board of Sapienza, University of Rome. Participants were informed of the general purpose of the study and their rights to confidentiality and to withdraw at any time without prejudice.
Measures
Except for the measure of NES use, all measures were administered on the first occasion of data collection. Measures were developed for each target behavior (i.e., study and NES use) and for each target population according to published guidelines for component theories of the integrated model (e.g., Girelli et al., 2016; Jacobs et al., 2011). Furthermore, measures were adapted to the behavioral context of reference (i.e., studying and NES use). Specifically, except for students’ motivation toward studying, Self-regulated strategies for Learning Efficacy (SLE; i.e., referred to studying) and action/coping planning about NES use, all TPB belief measures were formulated and administered for both studying and NES use. Finally, when necessary, Italian versions of the instruments were translated by two English-Italian bilinguals using standardized back translation procedures (Hambleton & Patsula, 1998). A full description of the measures is provided in the online supplemental materials (https://osf.io/ngcas/, Appendix B).
Autonomous Motivation
The Italian version of the academic motivation scale was used to measure the relative degree of students’ autonomous motivation toward studying (Alivernini & Lucidi, 2008; Vallerand et al., 1992). The scale consisted of five sets of items measuring students’ motivation, external regulation, introjected regulation, identified regulation, and intrinsic motivation toward studying and learning. Item responses were made on a 7-point Likert scale (1 = do not agree and 7 = totally agree) and, to maximize the parsimony of the proposed model; item scores were aggregated into a single relative autonomy index score (RAI; Ryan & Connell, 1989). This score represented a weighted aggregate of the five subscales scores and measured students’ relative degree of autonomous academic motivation (i.e., the RAI ranged from 2 to −2, with higher scores indicating relatively stronger autonomous motivation or, inversely, lower controlled motivation).
Self-Regulated Strategies for Learning Efficacy
Self-regulated strategies for Learning Efficacy (SLE) were measured using an adapted version of the scale (Zimmerman et al., 1992). This scale included nine items measuring students’ perceived confidence to use a variety of self-regulated learning strategies. Item responses were provided on 5-point Likert-type scales (1 = not well at all and 5 = very well).
Theory of Planned Behavior
Measures from the TPB were developed based on the recommendations of Ajzen (1991) and prior research (e.g., Girelli et al., 2016). In all cases, item scores were aggregated to produce a single scale score for each variable. The measure of attitudes was measured on six 7-point semantic differential scales using bipolar adjectives (e.g., “interesting–boring”). SN was measured using three items with students’ responses collected on 7-point Likert-type scales (1 = strongly disagree and 7 = strongly agree). PBC was measured by three items with responses collected on 7-point Likert-type scales (1 = strongly disagree and 7 = strongly agree). Finally, students’ intentions were measured using three items with responses collected on a 7-point Likert-type scale (1 = strongly disagree and 7 = strongly agree).
Planning
Students’ action planning and coping planning to use NES were measured by four items, each based on Sniehotta and colleagues’ (2005) scale. Responses were provided on 7-point Likert-type scales (1 = not true at all and 7 = very true).
Neuro-Enhancement Substances Use
The target behavior was measured on both occasions by asking participants to self-report their NES use over the last four months. This assessment focused on prescription drugs, illicit drugs, and/or “soft-enhancers”. Participants were also asked if they had a specific illness-related prescription for the drugs they reported using. For all substance types, participants reported their responses on a 3-point scale ranging from yes, yes, but not in the last 4 months, or no. The behavioral measure was computed by aggregating scores across types of drugs into a binary scale (i.e., 1 = yes and yes, but not in the last four months; 0 = no).
Data Analysis
The longitudinal data were analyzed by employing VB-SEM (known as Partial Least Squares analysis), which was performed with the WARP PLS v.6.0 statistical software (Kock, 2017). VB-SEM is like a covariance-based SEM analysis in that it explicitly models measurement error through the construction of latent factors. However, unlike covariance-based SEM, VB-SEM estimates models using ranked data and is, therefore, distribution-free. Model estimation is less likely to be affected by model complexity, sample size, or deviations of the variable distributions from normality. VB-SEM analysis permits the evaluation of the model at the measurement level and at the structural level according to published criteria for VB-SEM models. At the measurement level, VB-SEM establishes construct validity of the latent factors using the average variance extracted (AVE) and the composite reliability coefficients (ρ), which should exceed .50 and .70, respectively. Discriminant validity is supported when the square root of the AVEs for each latent variable exceeds its correlation coefficient with other latent variables (Esposito Vinzi, 2010). At the structural level, VB-SEM estimates the overall adequacy of the set of hypothesized relations among the model constructs using the goodness-of-fit (GoF) index given by the square root of the product of the AVE and average R2 for the model with values of .100, .250, and .360 correspond to small, medium, and large effect sizes for model fit, respectively (Tenenhaus et al., 2005). Further information on the adequacy of the model is provided by the average path coefficient (APC) and average R2 (ARS) coefficients, both of which should be statistically significantly different from zero. Furthermore, the level of multicollinearity is estimated using the full collinearity variance inflation factor (AFVIF), with values lower than 3.300 indicative of no issues with multicollinearity. Missing data were imputed using linear regression interpolation as recommended (Kock, 2014).
A WARP PLS power analysis was conducted for each sample. The minimum sample size generated for both samples was n = 146, and the criteria that produced this estimate were (a) a minimum absolute significant path coefficient of .21, with the significance level used for hypothesis testing of p = .05 and (b) a power level of .80 (Kock, 2017).
The first series of VB-SEM analyses evaluated the measurements’ psychometric and constructed characteristics, whereas the second series of VB-SEM analyses evaluated the relations illustrated in Figure 1. Concerning the latter analyses, at the first stage, two VB-SEM analyses for each sample estimated the relations, respectively, without and with the predictive effects of time 1 NES use on the key variables and on time 2 NES use. This permitted us to test the extent to which behavioral stability over time attenuated the hypothesized effects. Pending adequate fit of the proposed model, the second stage of VB-SEM analyses evaluated model invariance, that is, the hypothesis that model relations would be statistically equivalent in both student groups. Multi-group invariance was considered for both measurements and structural relations (Kock, 2014), and these analyses provided estimates of the difference in parameters and confidence intervals about the difference. In addition, effect sizes (Cohen’s d) for the difference in the effects across samples were also calculated, along with the 95% confidence interval for each. Details about data files and analysis output are available on the online supplemental materials (https://osf.io/ngcas/, see “Data Files” and “WARP Analysis Output Files”).
Results
Preliminary Analyses
Composite reliability coefficients (ρ), scale reliability (α), AVE, and latent variable correlations are presented in the supplemental materials (https://osf.io/ngcas/, Appendix C, Tables C1 and C2). The coefficients indicated acceptable internal consistency for constructs (ρ range = .62 to .96), except for high school students’ PBC toward NES use (ρ = .42). In this latter case, one item with a low item-total correlation (“Using NES to increase my academic performance over the next 4 months depends…” answered from 1 = mainly on other people to 7 = mainly on me) was eliminated, resulting in improved measurement reliability (ρ = .64). AVE estimates also indicated adequacy of the latent variables (AVE range = .42 – .88). Factor intercorrelations for all measures suggested no problem with discriminant validity and collinearity.
Model Tests
Figures 2 and 3 summarize the path coefficients of the proposed models. Overall, the pattern of estimated effects exhibited good fit with the data according to the multiple fit and quality indices adopted for the high school (GoF = .321; APC = .184, p < .001; ARS = .156, p < .001; AVIF = 1.244) and university students (GoF = .305; APC = .163, p < .001; ARS = .129, p < .001; AVIF = 1.209) student sample.

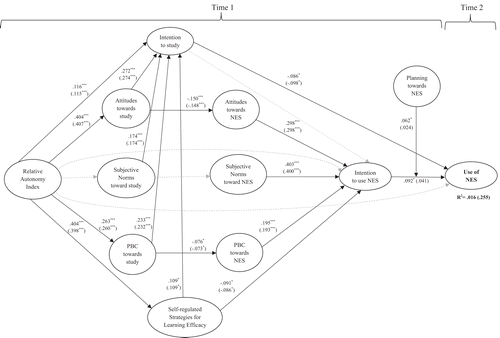
Students’ autonomous motivation had positive and statistically significant effects on their TPB beliefs concerning studying (H1a) and their SLE (H1b). The only exception was a null effect of university students’ autonomous motivation on SN for studying. Studying-related attitudes, SN, PBC (H2), and academic SLE (H5a), were positively related to students’ intention to study. The only exception was a null effect of attitude on the intention of high school students.
The analyses related to the relation between TPB constructs about studying, and TPB constructs about the use of NES supported our hypotheses (H3) and showed that students who had relatively stronger attitudes and perceived control about studying were less likely to endorse NES use. Furthermore, students’ SLE negatively influenced students’ intentions to use NES (H5b), but only among university students. Finally, TPB constructs about NES use were significantly and positively related to intentions to use NES (H4).
NES intentions predicted higher self-reported NES use (H6a), whereas intentions to study negatively predicted NES use (H6b). However, the latter effect held only among university students. Finally, planning moderated the relation between NES intention and NES use (H7), but only among university students.
In contrast to prediction, autonomous motivation to study did not directly predict intention to use NES (H8a) and NES use (H8b). Moreover, results indicated that relatively weaker attitudes toward studying indirectly predicted relatively stronger intentions to use NES by also increasing the chances of stronger attitudes toward NES use (H9). Furthermore, autonomous motivation indirectly influenced students’ intention to study by exerting predictive effects on some of the TPB variables concerning studying (H11a) and SLE (H11b). Details of indirect effects are provided in the supplemental materials (https://osf.io/ngcas/, Appendix D, Tables D1, D2, and D3).
Finally, the effects remained largely unchanged after controlling for the effects of past NES use. There were two exceptions to this finding: the effect of NES intentions on students’ NES use and the moderation effect of planning, which were smaller and no longer statistically significant when past NES use was included in the model. As expected, the inclusion of past NES use led to an increase in the explained variance in NES use accounted for by the model, both in high school (R2change = .18) and university students (R2change = .24).
Invariance of Model Effects Across Samples
We use the multi-group analyses to test the hypothesis that the model’s latent relations would be equivalent across student samples. These analyses were legitimate considering the invariance found at the measurement level (i.e., students of both groups assigned the same meaning to measures). Most of the paths in the model were invariant across groups, and there were only a few differences in parameter estimates across samples (H12, see Table 1). Effect sizes for all significant differences in parameter estimates were small-to-medium in size (range = .11–.27).
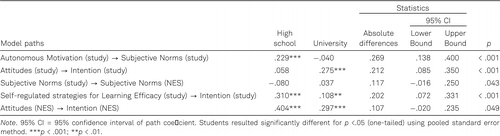
Discussion
The study adopted the general view that students who assign personal value to studying and knowledge acquisition and freely choose to pursue their studying goals and efforts would hold positive views about studying and disregard any alternative means to academic success. Empirically, the study tested the hypothesis that autonomously motivated students would hold positive beliefs and intentions about studying, and these, in turn, would render positive views, intentions, and possible use of NES less likely.
The research relied on data collected from high school and university students and examined a longitudinal model of effects in which the key outcome was students’ retrospective reports of their possible NES use. Well-fitting SEM supported the proposed model effects in both samples, with few exceptions. As such, the findings support and extend previous research, especially in academic contexts (e.g., Judson & Langdon, 2009).
The study was novel in several respects. It contemplated the notion that studying and NES use is distinct and yet related behavioral domains. Autonomously motivated students were more likely to bring their sets of beliefs in line with their motives. Such alignment is strategic, as these beliefs are proposed as the immediate determinants of future action, and findings suggest that individuals mobilize their beliefs to pursue autonomously motivated behaviors in the future. Therefore, and not surprisingly, students’ autonomous motivation, stronger attitudes, SN, and PBC beliefs concerning studying were negatively related to NES use. In other words, lower autonomous motivation to study led some students to endorse NES use more strongly and choose the use of NES, perhaps as an aid to enhance their performance.
The study also considered students’ past NES use as an essential means to evaluate the sufficiency of the model. As expected, past NES use predicted most of the variables in the model and accounted for substantial variance in students’ later self-reports of NES use, leading to some clear attenuation of model effects. Nevertheless, most model variables still accounted for unique variance in students’ intention to study, their intention to use NES, and their NES use. These longitudinal findings are consistent with existing social cognition research and with integrated models (Hagger et al., 2018), as they strongly support the notion that past behavior attenuates, but does not extinguish, model effects, that is, our guiding model is sufficient in accounting for unique variance.
Not surprisingly, students’ past NES use had its strongest effect on later NES use, increasing explained variance in the behavioral outcome by more than half. Theorists have suggested that the pervasive effects of past behavior may account for habitual effects (Hagger et al., 2018). Although past behavior is not a measure of habit, it may reflect habitual influences (Ouellette & Wood, 1998), and research has suggested that variables representing habit as a construct mediate past behavior effects (Brown et al., 2020; Hagger et al., 2018), supporting this claim. This should not come as a surprise for NES use, given that individuals who take NES are likely to do so in similar contexts and respond to similar cues (e.g., in the run-up to exams when there is a need to study). Given that habits tend to be built up through consistent behavioral responses in the face of consistent cues, it might be that participants develop strong habits for NES use. We can only infer these habitual effects because we did not directly measure NES habits, and that may very well be an avenue for future research (Hagger, 2019). If the effects of past behavior were mediated by a measure of habit, then we would have sharp confirmation of the hypothesis that past behavior effects represent habits.
The current study innovatively hypothesized a moderation effect of planning on the intention-behavior relation for NES use, consistent with previous research (Gollwitzer, 1999) and with proposals of previous integrated models (Hagger & Chatzisarantis, 2014). University students who had formed clear plans to take NES were more likely to enact their intentions. Notably, effect sizes were almost identical, suggesting that the effect might be present but modest in size. Thus, while supporting the notion that planning might be part of the decision-making process in the enactment of NES intentions, findings also suggested that planning might not be that pertinent or pervasive. This effect was further attenuated by the inclusion of past behavior. This suggests that habitual NES use obviates the need for planning. However, it would be interesting to assess whether students with strong or weak habits for NES use would be less likely to form plans for NES consumption.
We also examined the invariance of effects, whether processes and their effects held among high school and university students. One of the key assumptions of existing motivational and social cognitive research is that modeled effects represent universal and generalized processes holding across behaviors, contexts, and populations. Thus, effects’ variations would be in magnitude rather than whether they exist.
Our multi-group analyses showed remarkable consistency in the presence and magnitude of effects across samples, with very few differences in the relative strength of the model effects. For university students, their attitudes about studying had a relatively greater influence on their intentions to study than their self-efficacy. For high school students, instead, self-efficacy, but not attitudes about studying, influenced students’ intentions to study. There might be two reasons for these differences. At this moment, these reasons are merely speculative as, to the best of our knowledge, there is no research addressing this specific issue. First, it is possible that self-efficacy or personal confidence is more pertinent and paramount to high school students when it comes to studying for exams, as the context of high school calls for several abilities across several study subjects. For university students, their attitudes might be more important, perhaps because they see the advantages or values of what they have chosen to study. A second related reason might be that university students focus on specific subjects (majors and minors) rather than general education. For them, therefore, a general measure of self-efficacy, like the one adopted in the study, might have been less robust in capturing differences in personal confidence. Of course, such speculations should or might be corroborated in future research that, for instance, assesses students’ actual abilities and/or subject-specific self-efficacy.
Limitations
Several limitations should be noted. First, there are limits in terms of the generalizability of the findings to student populations, as the study samples were not randomly recruited stratified samples. Second, although the adoption of behavioral self-reports is quite common in behavioral research, the study would have benefitted from the adoption of more comprehensive measures, such as diaries or tracking NES use by ecological momentary assessment (e.g., daily monitoring of target behaviors using online apps). Third, we considered the different types of NES, combining them in a unique category to increase the power of statical testing concerning the guiding integrated model. Future studies might benefit from analyzing separately the different classes of substances to take into consideration the distinct characteristics of each category of NES. Moreover, the study did not include measures of implicit attitudes and habits toward studying and NES use. Future research should incorporate implicit constructs as predictors alongside the motivational and social cognition constructs used in the current model (Hagger, 2019; Hagger & Chatzisarantis, 2014).
Conclusions
The study findings may inform future interventions to curb NES use and promote better health among high school and university students. Prior similar research (e.g., Reeve et al., 2020) indicated that motivational and social cognitive factors could be the target of intervention programs. In line with the present findings, future interventions may thus challenge students’ views about NES use and NES pervasiveness in studying, as well as promote students’ personal confidence through appropriate goals and school experiences.
1Scholars identified three classes: prescription drugs include stimulant drugs usually prescribed to treat attention deficits and sleep disorders (e.g., methylphenidate); drugs of abuse include alcohol and illicit drugs such as opiates like cocaine; “soft” enhancers include foods and beverages containing caffeine, food supplements, and nicotine (Maier & Schaub, 2015).
References
1991). The theory of planned behavior. Orgnizational Behavior and Human Decision Processes, 50(2), 179–211. https://doi.org/10.1016/0749-5978(91)90020-T
(2008). The academic motivation scale (AMS): factorial structure, invariance, and validity in the Italian context. TPM – Testing, Psychometrics Methodology in Applied Psychology, 15(4), 211–220.
(2020). The mediating role of constructs representing reasoned-action and automatic processes on the past behavior-future behavior relationship. Social Science and Medicine, 258(February), Article
(113085 . https://doi.org/10.1016/j.socscimed.2020.1130852007). Neuropsychiatric effects of prescription drug abuse. Neuropsychology Review, 17(3), 363–380. https://doi.org/10.1007/s11065-007-9037-7
(2012). Self-determined forms of motivation predict sport injury prevention and rehabilitation intentions. Journal of Science and Medicine in Sport, 15(5), 398–406. https://doi.org/10.1016/j.jsams.2012.03.016
(2020). Reciprocal relations between autonomous motivation from self-determination theory and social cognition constructs from the theory of planned behavior: A cross-lagged panel design in sport injury prevention. Psychology of Sport and Exercise, 48, Article
(101660 . https://doi.org/10.1016/j.psychsport.2020.1016601985). Intrinsic motivation and self-determination in human behavior. Plenum Press.
(2000). The “what” and “why” of goal pursuits: Human needs and the self-determination of behavior. Psychological Inquiry, 11(4), 227–268. https://doi.org/10.1207/S15327965PLI1104_01
(2020). Psychostimulant use for neuroenhancement (smart drugs) among college students in Brazil. Substance Use and Misuse, 55(4), 613–621. https://doi.org/10.1080/10826084.2019.1691597
(2013). An examination of prescription stimulant misuse and psychological variables among sorority and fraternity college populations. Journal of Attention Disorders, 17(2), 87–97. https://doi.org/10.1177/1087054711428740
(2010). Handbook of partial least squares: Concepts, methods and applications. Springer.
(2014). Substances used and prevalence rates of pharmacological cognitive enhancement among healthy subjects. European Archives of Psychiatry and Clinical Neuroscience, 264(1), 83–90. https://doi.org/10.1007/s00406-014-0537-1
(2022). Data and supplemental materials for “Identifying determinants of neuro-enhancement substances: Application of an integrated theoretical model.” https://osf.io/ngcas/
(2016). From perceived autonomy support to intentional behaviour: Testing an integrated model in three healthy-eating behaviours. Appetite, 96, 280–292. https://doi.org/10.1016/j.appet.2015.09.027
(1999). Implementation intentions strong effects of simple plans. American Psychologist, 54(7), 493–503. http://kops.uni-0000000konstanz.de/bitstream/handle/123456789/10101/99Goll_ImpInt.pdf?sequence=1&isAllowed=y
(1997). Implementation intentions and effective goal pursuit. Journal of Personality and Social Psychology, 73(1), 186–199. https://doi.org/10.1037/0022-3514.73.1.186
(2008). Towards responsible use of cognitive-enhancing drugs by the healthy. Nature, 456(7224), 872. https://doi.org/10.1038/456872a
(2009). Theoretical integration in health psychology: Unifying ideas complementary explanations. British Journal of Health Psychology, 14(2), 189–194. https://doi.org/10.1348/135910708X397034
(2019). Habit and physical activity: Theoretical advances, practical implications, and agenda for future research. Psychology of Sport & Exercise, 42, 118–129. https://doi.org/10.1016/j.psychsport.2018.12.007
(2016). Using meta-analytic path analysis to test theoretical predictions in health behavior: An illustration based on meta-analyses of the theory of planned behavior. Preventive Medicine, 89, 154–161. https://doi.org/10.1016/j.ypmed.2016.05.020
(2009). Integrating the theory of planned behaviour and self-determination theory in health behaviour: A meta-analysis. British Journal of Health Psychology, 14(2), 275–302. https://doi.org/10.1348/135910708X373959
(2014). An integrated behavior change model for physical activity. Exercise and Sport Sciences Reviews, 42(2), 62–69. https://doi.org/0091-6331/4202/62Y69
(2018). Motivational predictors of students’ participation in out-of-school learning activities and academic attainment in science: An application of the trans-contextual model using Bayesian path analysis. Learning and Individual Differences, 67, 232–244. https://doi.org/10.1016/j.lindif.2018.09.002
(2014). Autonomous and controlled motivational regulations for multiple health-related behaviors: Between- and within-participants analyses. Health Psychology and Behavioral Medicine, 2(1), 565–601. https://doi.org/10.1080/21642850.2014.912945
(2014). Implementation intention and action planning interventions in health contexts: State of the research and proposals for the way forward. Applied Psychology: Health and Well-Being, 6(1), 1–47. https://doi.org/10.1111/aphw.12017
(2018). The reasoned action approach applied to health behavior: Role of past behavior and tests of some key moderators using meta-analytic structural equation modeling. Social Science and Medicine, 213(1), 85–94. https://doi.org/10.1016/j.socscimed.2018.07.038
(2017). Predicting sugar consumption: Application of an integrated dual-process, dual-phase model. Appetite, 116, 147–156. https://doi.org/10.1016/j.appet.2017.04.032
(1998). Adapting tests for use in multiple languages and cultures 1. Social Indicators Research, 45(1), 153–171. https://doi.org/10.1023/A:1006941729637
(2011). Testing an integrated model of the theory of planned behaviour and self-determination theory for different energy balance-related behaviours and intervention intensities. British Journal of Health Psychology, 16(1), 113–134. https://doi.org/10.1348/135910710X519305
(2009). Illicit use of prescription stimulants among college students: Prescription status, motives, theory of planned behaviour, knowledge and self-diagnostic tendencies. Psychology, Health and Medicine, 14(1), 97–104. https://doi.org/10.1080/13548500802126723
(2020).
(Changing behavior using the model of action phases . In M. S. HaggerL. D. CameronK. HamiltonN. HankonenT. LintunenEds., The handbook of behavior change (pp. 77–88). Cambridge University Press. https://doi.org/10.1017/9781108677318.0062014). Advanced mediating effects tests, multi-group analyses, and measurement model assessments in PLS-based SEM. International Journal of E-Collaboration, 10(1), 1–13.
(2017). WarpPLS User Manual: Version 6.0. https://scriptwarp.com/warppls/
(2013). Cognitive enhancement in Canadian medical students. Journal of Psychoactive Drugs, 45(4), 360–365. https://doi.org/10.1080/02791072.2013.825033
(2018). Non-medical prescription stimulant use to improve academic performance among Australian university students: Prevalence and correlates of use. BMC Public Health, 18(1), 1–7. https://doi.org/10.1186/s12889-018-6212-0
(2015). The importance of stress, self-efficacy, and self-medication for pharmacological neuroenhancement among employees and students. Drug and Alcohol Dependence, 156, 221–227. https://doi.org/10.1016/j.drugalcdep.2015.09.012
(2013). To dope or not to dope: Neuroenhancement with prescription drugs and drugs of abuse among Swiss university students. PLoS One, 8(11), Article
(e77967 . https://doi.org/10.1371/journal.pone.00779672015). The use of prescription drugs and drugs of abuse for neuroenhancement in Europe: not widespread but a reality. European Psychologist, 20(3), 155–166. https://doi.org/10.1027/1016-9040/a000228
(2017). Brain doping: Stimulants use and misuse among a sample of Italian college students. Journal of Preventive Medicine and Hygiene, 58(2), E130–E140. http://www.ncbi.nlm.nih.gov/pubmed/28900353%0Ahttp://www.pubmedcentral.nih.gov/articlerender.fcgi?artid=PMC5584082
(2007).
(Health cognition assessment . In A. B. S. AyersC. McManusS. NewmanK. WallstonJ. WeinmanR. WestEds., Cambridge handbook of psychology, health and medicine (2nd ed., pp. 260–266). Cambridge University Press.2015).
(Theory of reasoned action, theory of planned behavior, and the integrated behavioral model . In K. GlanzB. K. RimerK. ViswanathEds., Health behavior and health education: Theory, research, and practice (pp. 95–124). Jossey-Bass.2012). Self-Determination Theory applied to health contexts: A meta-analysis. Perspectives on Psychological Science, 7(4), 325–340. https://doi.org/10.1177/1745691612447309
(1998). “Inclined abstainers”: A problem for predicting health-related behaviour. British Journal of Social Psychology, 37(2), 151–165. https://doi.org/10.1111/j.2044-8309.1998.tb01162.x
(1998). Habit and intention in everyday life: The multiple processes by which past behavior predicts future behavior. Psychological Bulletin, 124(1), 54–74. https://doi.org/10.1037/0033-2909.124.1.54
(2020). An autonomy-supportive intervention to develop students’ resilience by boosting agentic engagement. International Journal of Behavioral Development, 44(4), 325–338. https://doi.org/10.1177/0165025420911103
(2010). Modafinil and methylphenidate for neuroenhancement in healthy individuals: A systematic review. Pharmacological Research, 62(3), 187–206. https://doi.org/10.1016/j.phrs.2010.04.002
(1989). Perceived Locus of Causality and internalization: Examining reasons for acting in two domains. Journal of Personality and Social Psychology, 57(5), 749–761. https://doi.org/10.1037/0022-3514.57.5.749
(2000). Self-determination theory and the facilitation of intrinsic motivation, social development, and well-being. American Psychologist, 55(1), 68–78. https://doi.org/10.1037/0003-066X.55.1.68
(2005). Action planning and coping planning for long-term lifestyle change: Theory and assessment. European Journal of Social Psychology, 35(4), 565–576. https://doi.org/10.1002/ejsp.258
(2005). PLS path modeling. Computational Statistics and Data Analysis, 48(1), 159–205. https://doi.org/10.1016/j.csda.2004.03.005
(2011). The non-medical use of prescription drugs, policy direction issues. United Nations Office on Drugs and Crime. http://www.unodc.org/unodc/en/drug-prevention-and-treatment/non-medical-use-prescription-drugs.html
. (1992). The Academic Motivation Scale: A measure of intrinsic, extrinsic, and amotivation in education. Educational and Psychological Measurement, 52(4), 1003–1019. https://doi.org/10.1177/0013164492052004025
(2016). Non-medical prescription stimulant use in graduate students: Relationship with academic self-efficacy and psychological variables. Journal of attention disorders, 20(9), 741–753. https://doi.org/10.1177/1087054714529816
(2015). The complexity of neuroenhancement and the adoption of a social cognitive perspective. Frontiers in Psychology, 6, Article
(1880 . https://doi.org/10.3389/fpsyg.2015.018801992). Self-motivation for academic attainment: The role of self-efficacy beliefs and personal goal setting. American Educational Research Journal, 29(3), 663–676. https://doi.org/10.3102/00028312029003663
(